Halicin Antibiotic — A Tale of Discovery and Artificial Intelligence
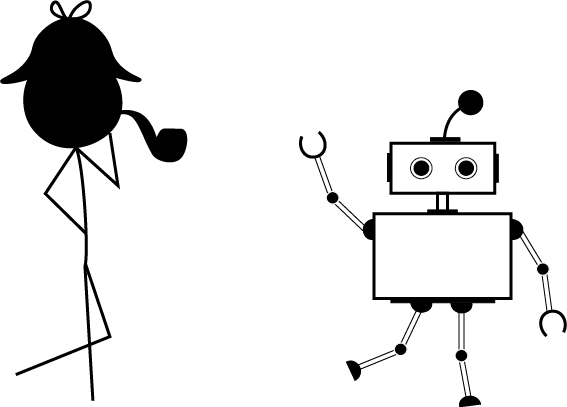
Discovering new antibiotics easy… someday… long ago. However, things changed drastically. To date, the process of discovering new drugs that are effective against pathogenic bacteria is (also because of the rise of new antibiotic-resistance mechanisms) time and money consuming — and hardly ever efficient.
Time to ask for the greatest detective of all time to take care of this.
Common screening techniques are based on the cultivation of environmental microbes. If this step is successful (which is not guaranteed as approximately 99 % of all microorganisms just will not grow in the lab), isolates or their metabolic products are transferred onto petri-dishes that are covered with another bacterial species. Thereafter, scientists look for areas on these petri-dishes where there was no bacterial growth — a so-called inhibition zone.
Doesn’t seem too complex, does it? Well, it isn’t. However, there is this one downside to why no scientist is getting excited because they inhibited bacterial growth by using metabolic products of other microorganisms: More often than not, you’re only discovering something that is already known of.
Because of discovering the same substances over and over again (and to avoid unsubstantiated excitement), smart brains came up with the idea of using databases to search for molecules that are similar to known antibiotics. However, there is always a downside. Screeing the incredible amount of databases filled with an uncountable number of chemical structures takes years at least.
Sherlock!
In the end, the molecules found in the sea of data need to be tested under real conditions. And as if this wouldn’t be enough, there is also the need to evaluate potential side effects. Sisyphus said to say hello!
However, computers and the so-called artificial intelligence (AI) are here to help us and a well-known tool when the in-house processing power is not enough.
You’ll figure it out, Sherlock.
A team of scientists from England recently were the first to use their computational power to let the computational power of their computers do the work of screening for new potential antibiotics (1). Computer scientists and biologists together developed an AI and fed it with 2000 structures of antimicrobial substances until it learned to differentiate between antibiotic and non-antibiotic molecules. Afterward, they used their trained AI to screen for ~11 000 molecules. As a result, they got 89 promising substances that potentially could inhibit bacterial growth. 89 out of 11 000. Have fun doing this by hand.
Halicin was one of the 89 molecules that looked especially promising on first (and second) sight. Initially, it has been developed to treat diabetes. However, Halicin failed but it is now getting a second chance as an antibiotic. First trials in the labs and on mice are very promising (2) as Halicin seems to have a bactericidal effect on many relevant human pathogens — including Acinetobacter baumanii, a pan-resistant superbug.
However, there is even more that makes it really likely, that Halicin will start a second career as an antibiotic. Its completely new mechanism of action (inhibiting a functional proton layer; a charged layer that covers that cell and is used for energy production) hardly provokes new resistances (so far). Therefore, depending on the culture media, no antibiotic resistance was observed after 7 or 30 days respectively. In comparison have a look at this video (3) to see how fast bacteria usually develop resistance mechanisms against other antibiotics.
The discovery of new antibiotics thanks to artificial intelligence is no longer science fiction as Halicin is showing impressively. Even though the global antibiotic (resistance) crisis is not only caused by the lack of new antibiotics, this technical tool can help by tackling the worldwide problem. Especially a far more attractive cost-benefit equation could lead to more pharmaceutical companies focusing on the development and discovery of new antibacterial substances.
Either way, Sherlock perhaps isn’t the greatest detective anymore…
References
(1) Stokes JM, Yang K, Swanson K, Jin W, Cubillos-Ruiz A, Donghia NM, MacNair CR, French S, Carfrae LA, Bloom-Ackermann Z, Tran VM, Chiappino-Pepe A, Badran AH, Andrews IW, Chory EJ, Church GM, Brown ED, Jaakkola TS, Barzilay R, Collins JJ. A Deep Learning Approach to Antibiotic Discovery. Cell. 2020 Feb 20;180(4):688–702.e13. doi: 10.1016/j.cell.2020.01.021. Erratum in: Cell. 2020 Apr 16;181(2):475–483. PMID: 32084340; PMCID: PMC8349178.
(2) Booq RY, Tawfik EA, Alfassam HA, Alfahad AJ, Alyamani EJ. Assessment of the Antibacterial Efficacy of Halicin against Pathogenic Bacteria. Antibiotics (Basel). 2021 Dec 2;10(12):1480. doi: 10.3390/antibiotics10121480. PMID: 34943692; PMCID: PMC8698312.
(3) Baym M, Lieberman TD, Kelsic ED, Chait R, Gross R, Yelin I, Kishony R. Spatiotemporal microbial evolution on antibiotic landscapes. Science. 2016 Sep 9;353(6304):1147–51. doi: 10.1126/science.aag0822 . PMID: 27609891 ; PMCID: PMC5534434.